Get to know the members of the HIDSS4Health Data Science Groups.
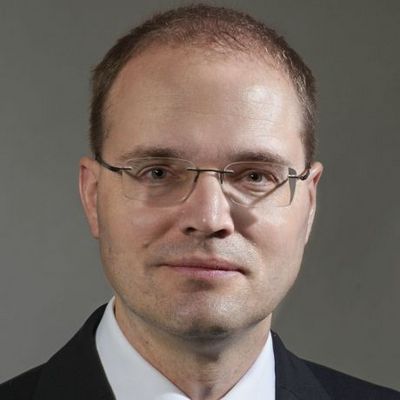
Achim Streit
KIT
Contact
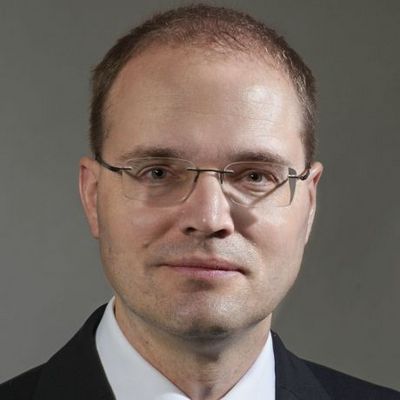
The Streit team is enabling data-intensive science through generic informatics R&D in the areas data management, data analytics, federated computing and scheduling.
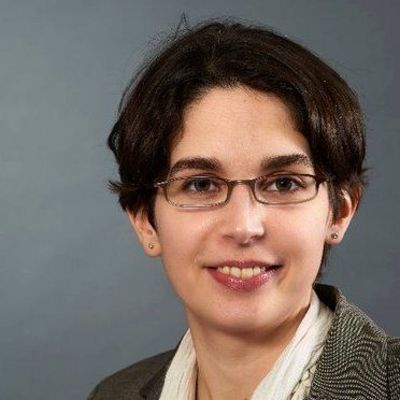
Anne Koziolek
KIT
Contact
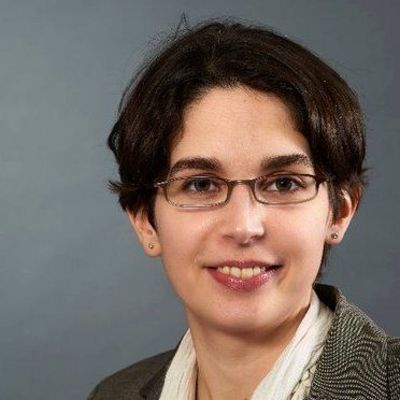
The Koziolek team researches how to ease development of data-intensive systems, esp. the selection of appropriate machine learning algorithms with respect to suitability and performance.
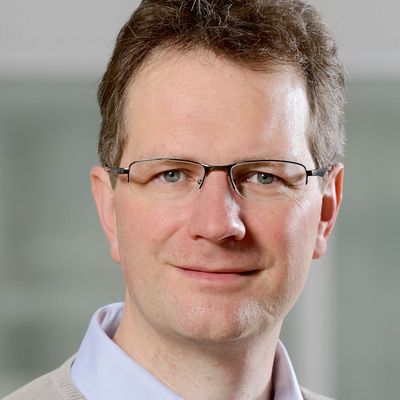
Benedikt Brors
DKFZ
Contact
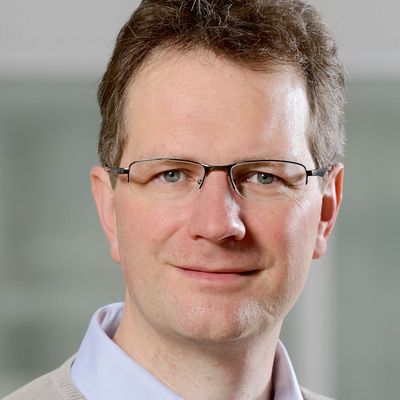
The Brors team develops algorithms for personalized medicine, cancer epigenetics and single-cell sequencing and applies them in a clinical context as well as in large international consortia.
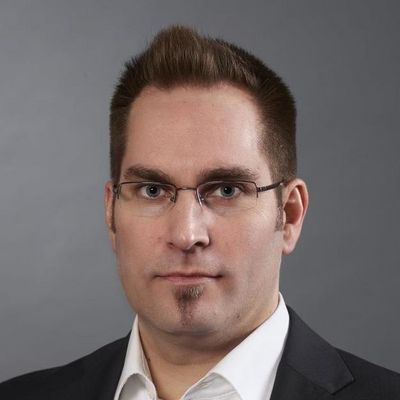
Carsten Dachsbacher
KIT
Contact
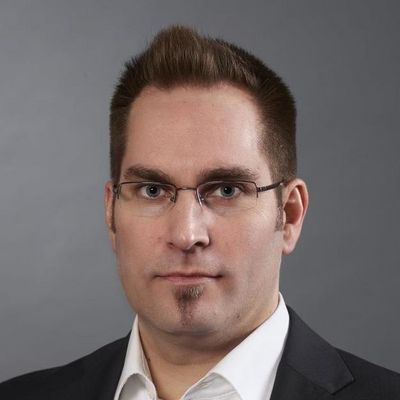
The research group developes methods for interactive visualization, high performance computer graphics, and radiative transport simulations.
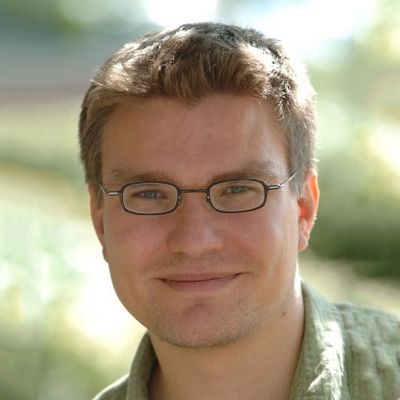
Carsten Rother
UNI HD
Contact
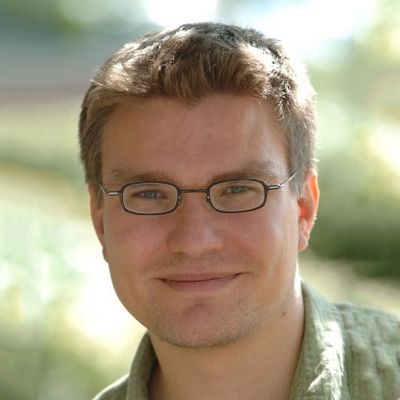
Carsten Rother received his diploma degree with distinction in 1999 from the University of Karlsruhe/Germany, and his PhD degree in 2003 from the Royal Institute of Technology (KTH) Stockholm/Sweden. From 2003 until 2013 he was researcher in the Computer Vision Group at Microsoft Research Cambridge/UK, lead by Andrew Blake. From 2014 until 2017 he was full Professor at TU Dresden/Germany. Since 2017 he is full Professor at Uni Heidelberg/Germany, heading the Computer Vision and Learning Lab. He is also coordinating director of the Heidelberg Collaboratory for Image Processing (HCI) and an AI consultant for companies. In the last years, two start-ups emerged from his lab https://rabbitai.de/ and https://www.copresence.tech/. His research interests are in the field of computer vision and machine learning, including related fields such as human-computer interaction and computational imaging.
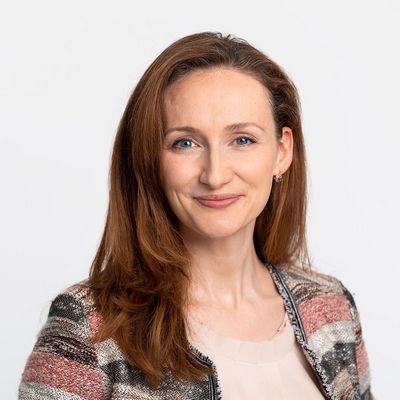
Emilia Grass
KIT
Contact
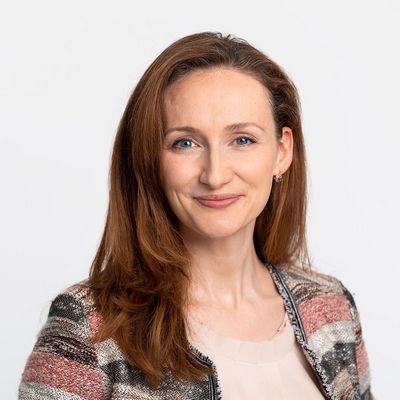
Our objective is to strengthen the resilience of healthcare systems against cyber-attacks by using stochastic programming, simulation and machine learning.
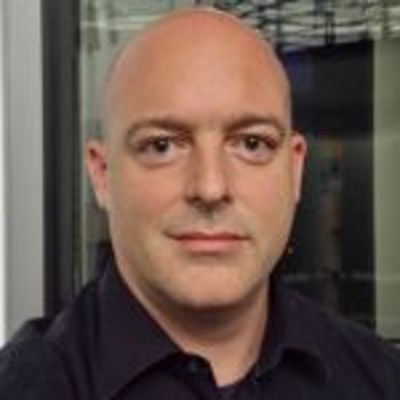
Filip Sadlo
UNI HD
Contact
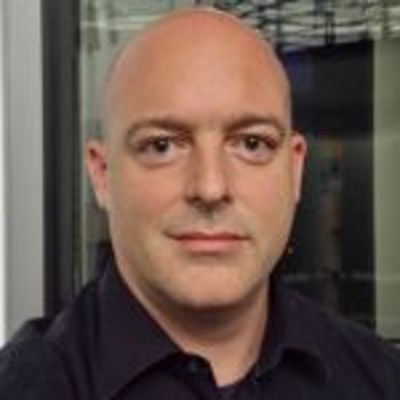
The Visual Computing Group develops novel techniques for visual data analysis, with a focus on topological analysis and feature extraction.
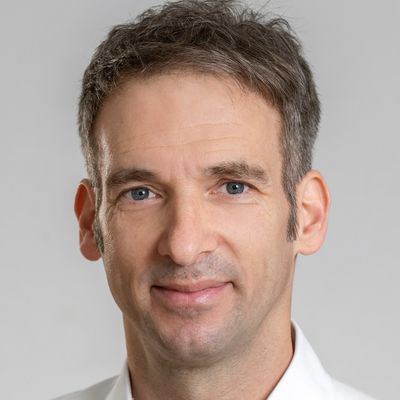
Holger Fröning
UNI HD
Contact
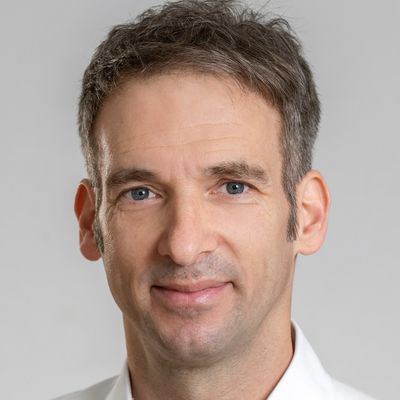
Performance, energy-efficiency and programmability: HPC/HPA, deep learning, reconfigurable logic. Example 1: deep learning on embedded systems. Example 2: simplified multi-GPU programming
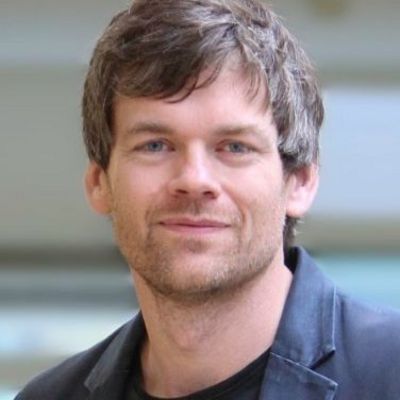
Klaus Maier-Hein
DKFZ
Contact
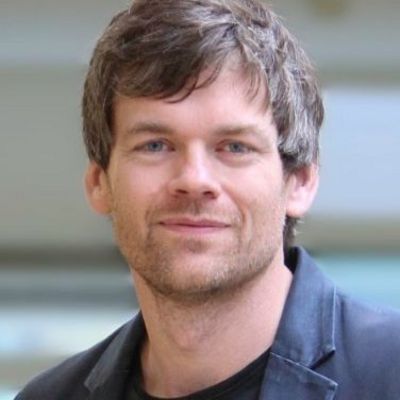
The Maier-Hein team develops machine learning algorithms, mathematical modelling approaches for computational image understanding and large-scale information processing.
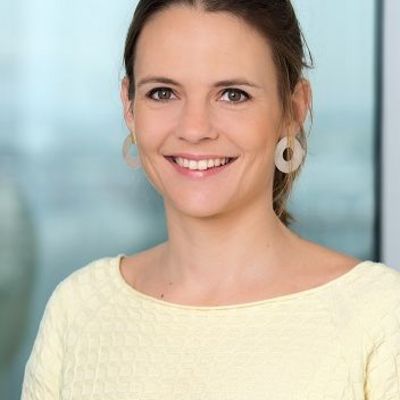
Lena Maier-Hein
DKFZ
Contact
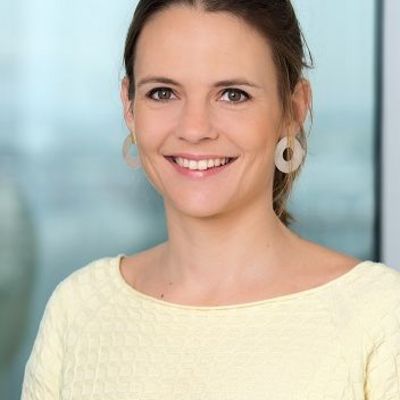
The Maier-Hein team aims to improve the quality of interventional healthcare and its value computationally. It supports the physician throughout the entire process of disease diagnosis, therapy and follow-up with the right information at the right time.
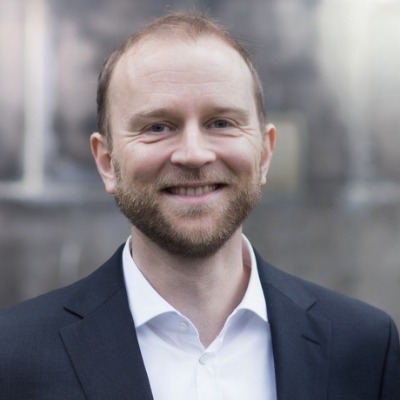
Martin Frank
KIT
Contact
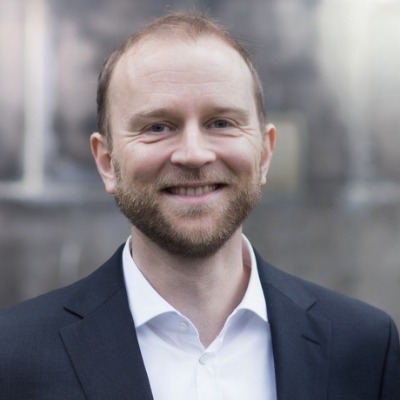
The research group Computational Science and Mathematical Methods (CSMM) is an interdisciplinary research group working on different challenges in mathematical modeling. We are unified by our interest in method-oriented mathematics, in mathematical modeling inspired by applications, and in the didactics of mathematical modeling.
Our research focuses on bringing modern mathematical techniques such as modeling, simulation, optimization, inverse problems, uncertainty quantification, and machine learning/artificial intelligence into the real-world. Both by teaching them to students in schools and universities and by advancing the research in relevant fields of application.
Furthermore, we take an interest in kinetic theory, developing models, numerical methods and software implementations and combining them with the aforementioned techniques.
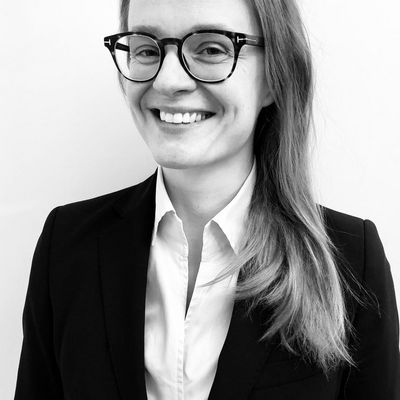
Melanie Schienle
KIT
Contact
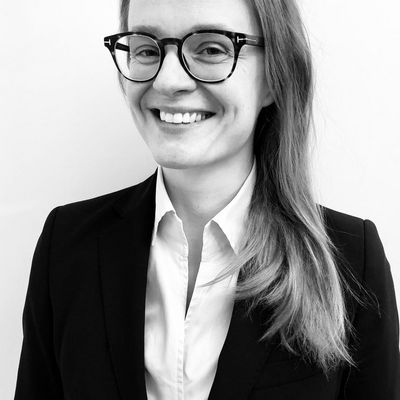
The Schienle team develops novel statistical methods for interpretable machine learning, including networks, treatment effects and prediction uncertainty.
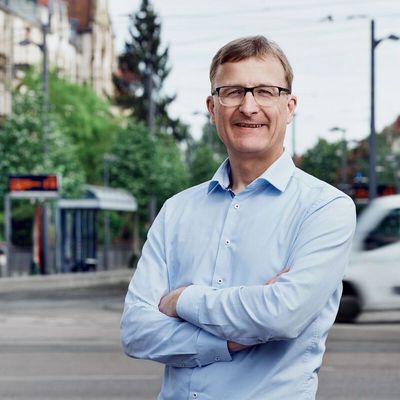
Michael Beigl
KIT
Contact
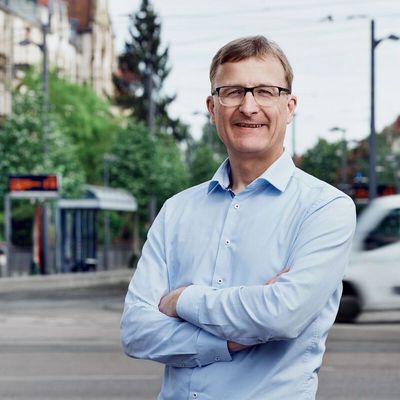
The Beigl team develops AI-driven analytics and Big Data methods and systems to solve problems in application domains.
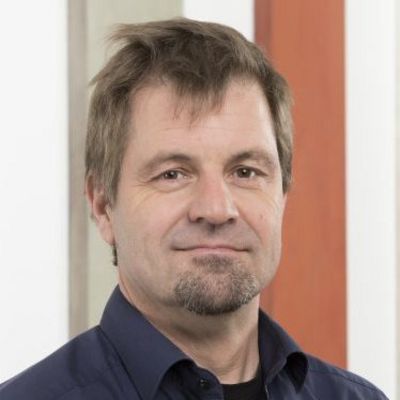
Michael Gertz
UNI HD
Contact
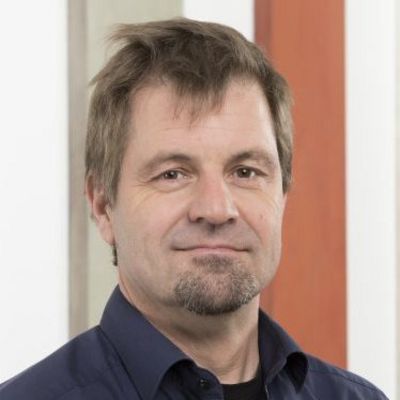
The Gertz team focuses on novel models and techniques in support of information extraction, data/text mining, machine learning, and network analysis for heterogeneous data.
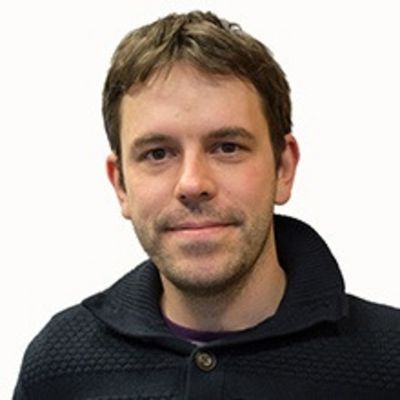
Oliver Stegle
DKFZ
Contact
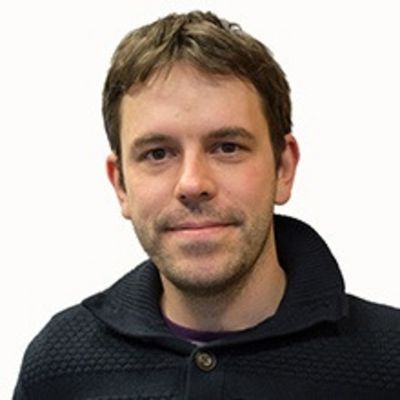
The Stegle group develops and applies statistical approaches and methods based on machine learning for analysing high-dimensional molecular data modalities.
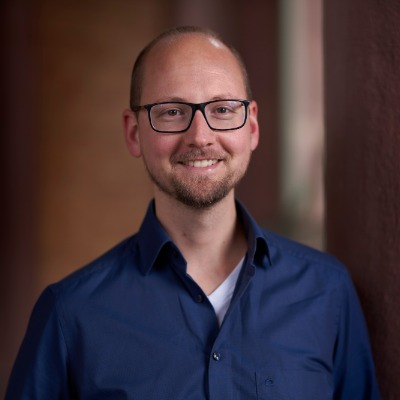
Pascal Friederich
KIT
Contact
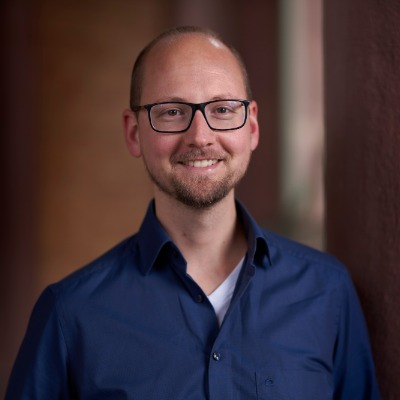
After his Ph.D. in physics, Pascal Friederich received a Marie-Sklodowska-Curie Postdoctoral Fellowship at Harvard University and the University of Toronto where he worked on machine learning methods for chemistry. In 2020 Pascal Friederich was appointed assistant professor at the Informatics Department of the Karlsruhe Institute of Technology, leading the AI for Materials Science (AiMat) research group. His research focuses on developing and applying machine learning methods for property prediction, simulation, understanding, and design of molecules and materials. In 2022, Pascal Friederich received the Heinz-Maier-Leibnitz Prize from the German Research Foundation.
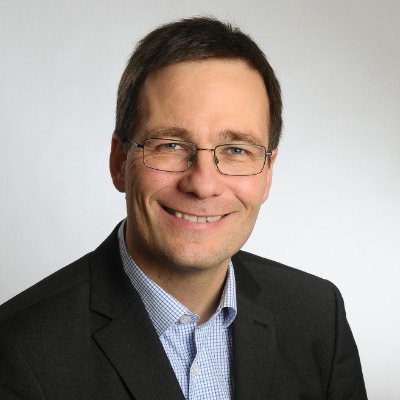
Peter Sanders
KIT
Contact
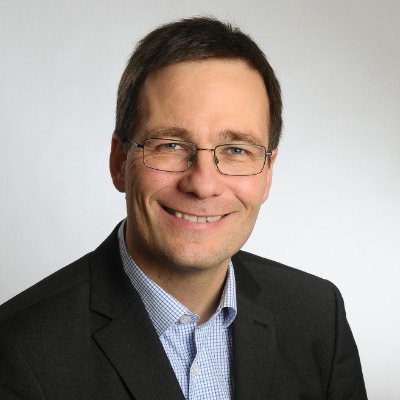
The Sanders team develops basic toolbox algorithms and software libraries for handling large data sets in a scalable way.
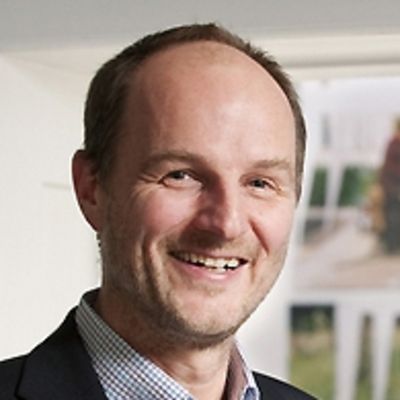
Rainer Stiefelhagen
KIT
Contact
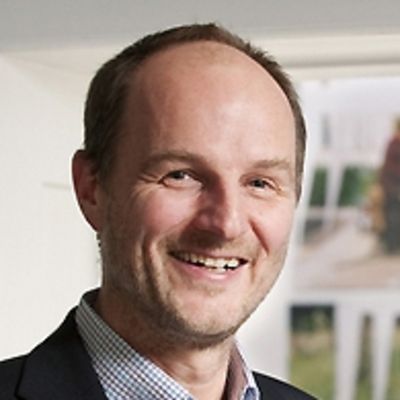
The Stiefelhagen team investigates methods to analyse images using weak supervision, for example by jointly analysing medical images and their associated clinical reports.
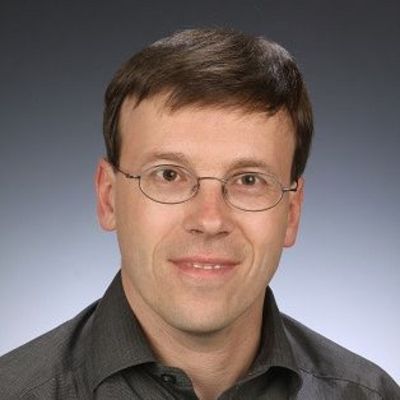
Ralf Mikut
KIT
Contact
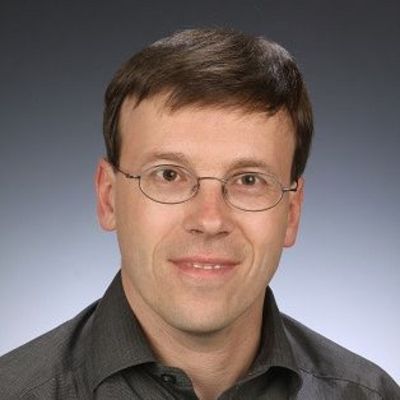
The Mikut team analyses 2D, 3D and 3D+t biomedical images and related data using image analysis and machine learning methods.
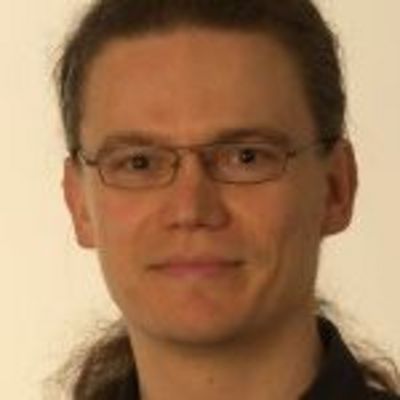
Robert Strzodka
UNI HD
Contact
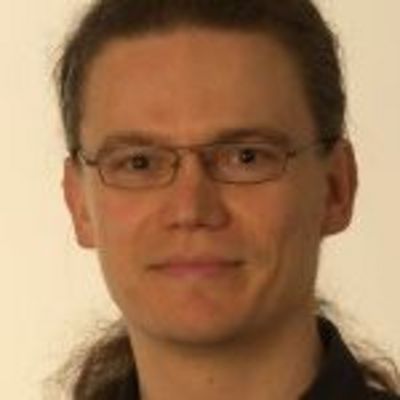
The chair Application Specific Computing focuses on efficient interactions of mathematic, algorithmic and architectural aspects in heterogeneous high performance computing (GPUs, FPGAs, many-core).
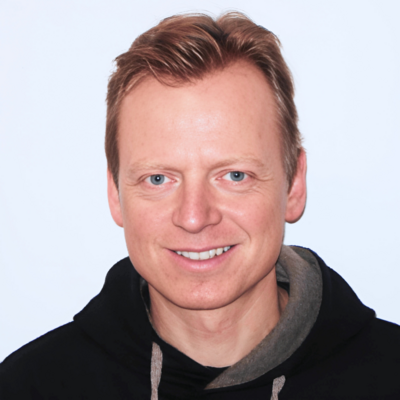
Stefan Riezler
UNI HD
Contact
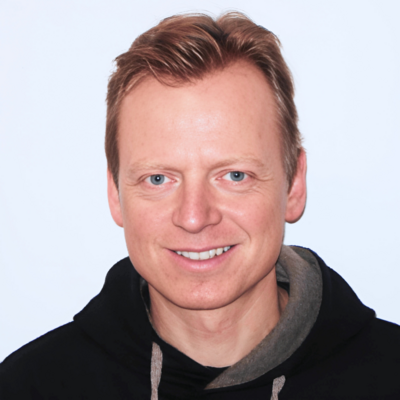
The Riezler group develops statistical machine learning methods for ambiguous and noisy data, with a focus on natural language processing.
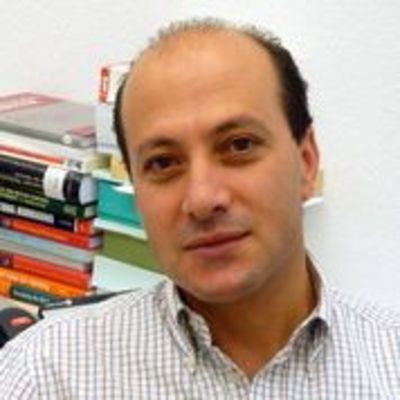
Tamim Asfour
KIT
Contact
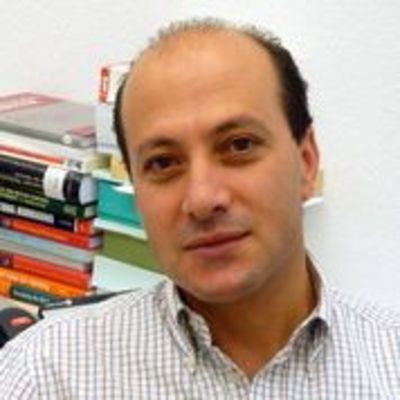
The Asfour team develop data-driven methods and algorithms for skill learning, motion generation and prediction in the context of robot-assisted surgery and exoskeletons.
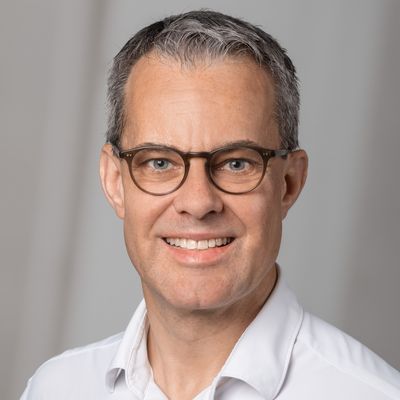
Till Bärnighausen
UNI HD
Contact
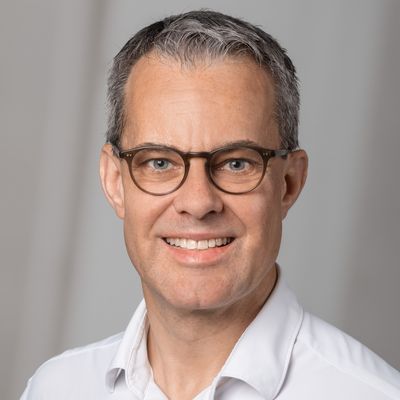
Till Bärnighausen is Alexander von Humboldt Professor and Director of the Heidelberg Institute of Global Health (HIGH), Heidelberg University, Germany. He is also Senior Faculty at the Africa Health Research Institute (AHRI) in South Africa and fellow at the Harvard Center for Population and Development Studies.
Till’s research focuses on creating and evaluating global health interventions. His research has been funded by the US National Institutes of Health, European Union, German Research Foundation, Alexander von Humboldt Foundation, Wellcome, Bill & Melinda Gates Foundation, Clinton Health Access Initiative, Else-Kröner-Fresenius Foundation, USAID, UNAIDS, WHO, KfW, World Bank.
Till has previously worked as professor at Harvard T.H. Chan School of Public Health; as medical doctor in Germany, China and South Africa; and as management consultant for McKinsey & Company. Till holds doctoral degrees in international and population health (Harvard) and history of medicine (Heidelberg), and master degrees in health systems management (LSHTM), financial economics (SOAS), and innovation and entrepreneurship (HEC Paris).
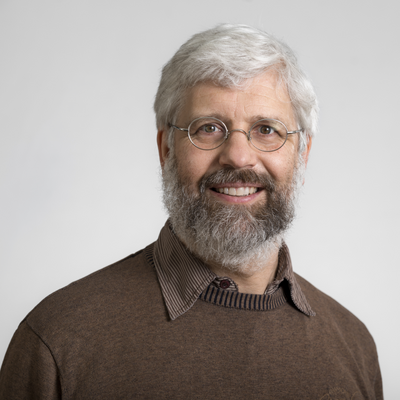
Ullrich Köthe
UNI HD
Contact
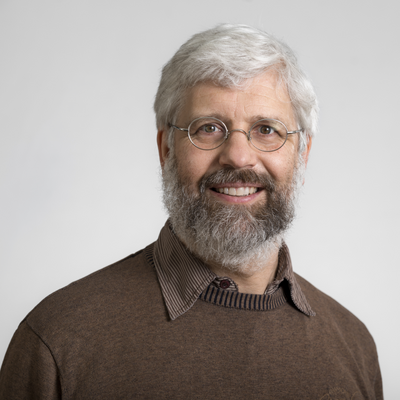
Ullrich Köthe’s team works on interpretable machine learning methods that help to extract knowledge from data in the natural and life sciences. Our speciality are invertible neural networks.
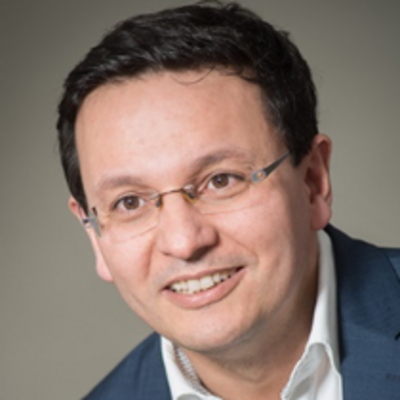
Vincent Heuveline
UNI HD
Contact
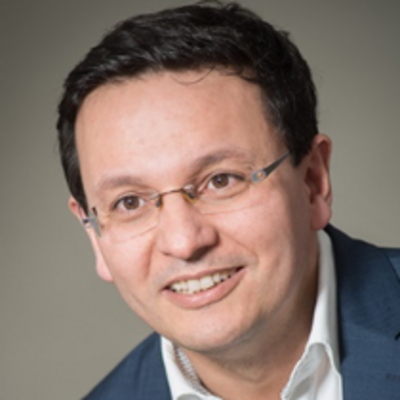
The EMCL focuses on uncertainty quantification (UQ) in scientific computing, high performance and data intensive computing, with main application focuses in medical engineering.
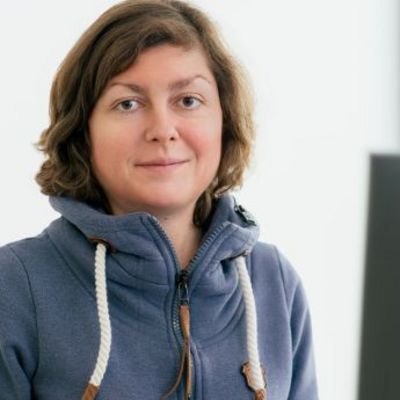
Nadja Klein
KIT
Contact
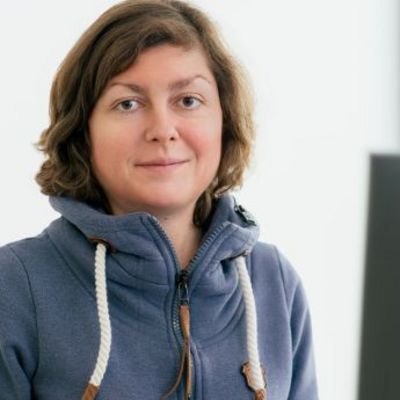
My research specializes in Bayesian learning, a powerful approach that allows to incorporate prior knowledge, quantify uncertainties, and bring insight to the “black boxes” of machine learning. By fusing the precision and reliability of Bayesian statistics with the adaptability of machine and deep learning, they aim to deliver the best of both worlds. More information about our activities can be found at https://kleinlab-statml.github.io/.
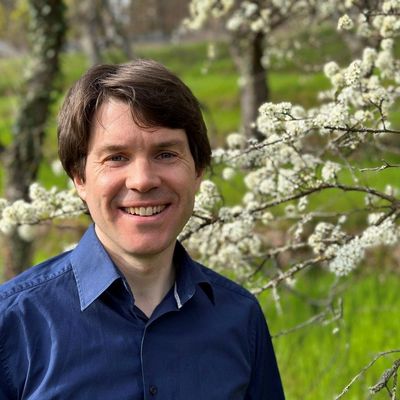
Andreas Ott
UNI HD
Contact
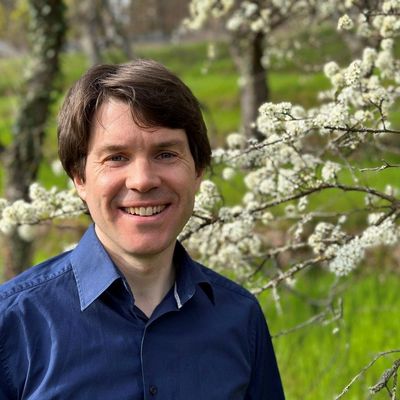
Geometry, topology and applications in the life sciences. I am currently working on the following topics:
- topological data analysis and computational topology
- geometric and topological methods in omics data analysis
- bounded cohomology and simplicial volume
- Higgs bundle moduli spaces, harmonic maps and representation varieties
- geometry of special functions (polylogarithms, hypergeometric functions)
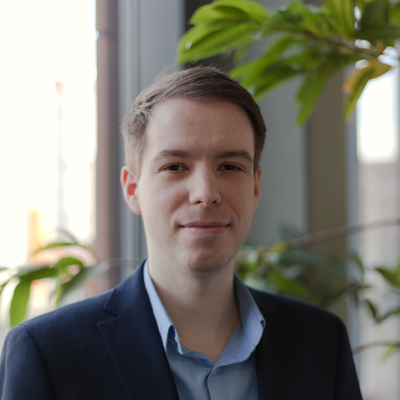
Scott Thiebes
KIT
Contact
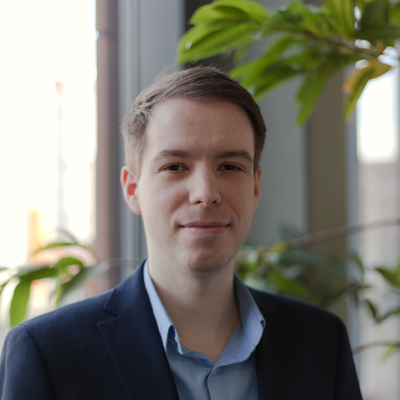
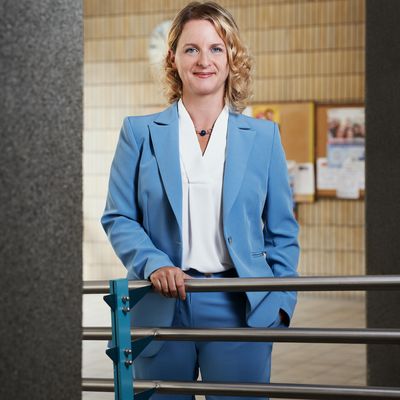
Maria Francesca Spadea
KIT
Contact
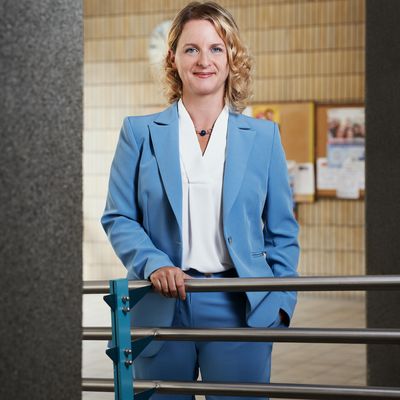
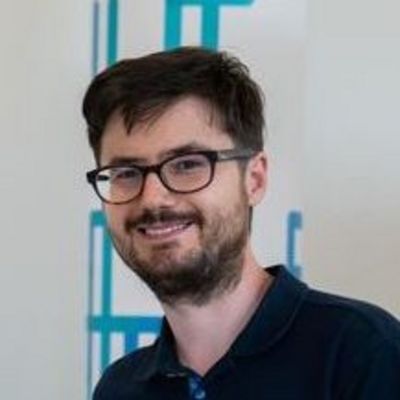
Gerhard Neumann
KIT
Contact
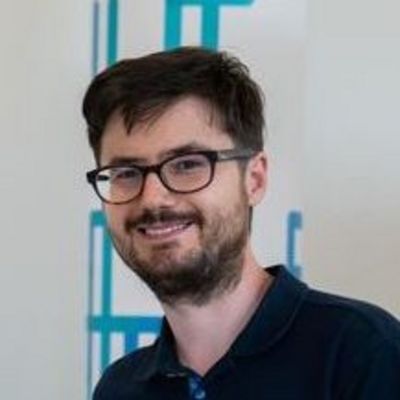
My research is therefore focused on the intersection of machine learning, robotics and human-robot interaction. My goal is to create data-efficient machine learning algorithms that that are suitable for complex robot domains. A strong focus of my research is to develop new methods that allow a human non-expert to intuitively teach a robot complex skills as well as to allow a robot to learn how to assist and collaborate with humans in an intelligent way. In my research, I always aim for a strong theoretical basis for my developed algorithms which are derived from first order principles. Yet, I also believe that an exhaustive assessment of the quality of an algorithm in a practical application is of equal importance.
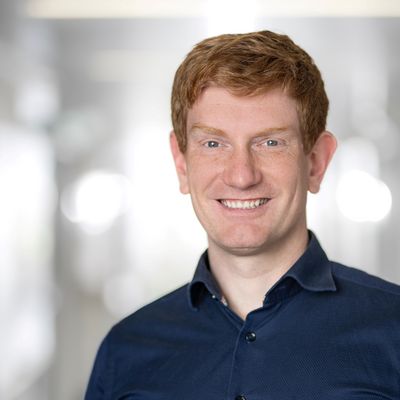
Axel Loewe
KIT
Contact
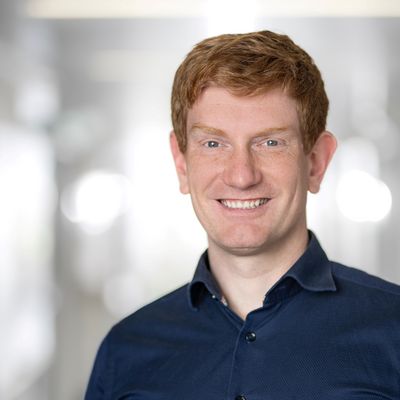
The Computational Cardiac Modeling group (head: Axel Loewe) aims at formulating the heart's function mathematically and realistically representing it with computational models. Our research is focused on the electrophysiology and mechanics of the heart, which we represent with multiscale models. These models include several biological levels of integration from ion channels via cells to tissues and organs and the relevant phenomena span a multitude of temporal and spatial scales (microseconds to minutes; micrometers to centimeters).
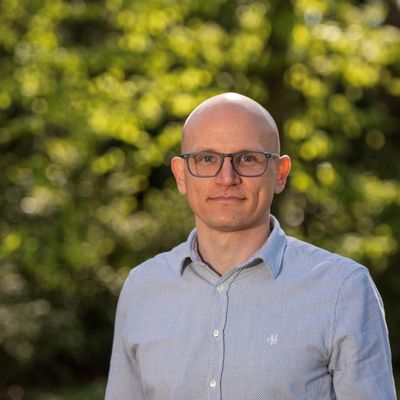
Sebastian Krumscheid
KIT
Contact
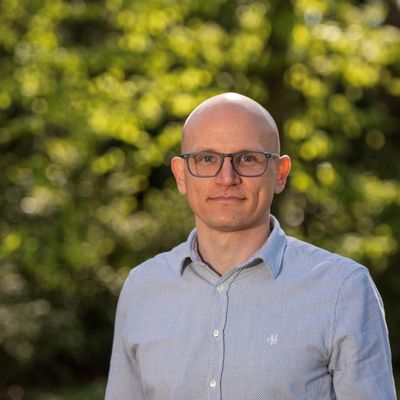
The Uncertainty Quantification (UQ) research group under the supervision of tenure-track Prof. Dr. Sebastian Krumscheid is located at the Scientific Computing Center (SCC) and is also associated with the Institute for Applied and Numerical Mathematics (IANM) of the KIT Faculty of Mathematics.
At the UQ group, we develop state-of-the-art mathematical and numerical techniques for the treatment and quantification of uncertainties at the interface of complex computational models and data. Our research activities focus on both theoretical and methodological aspects, as well as on interdisciplinary projects, where theoretically based methods are tailored to applications.
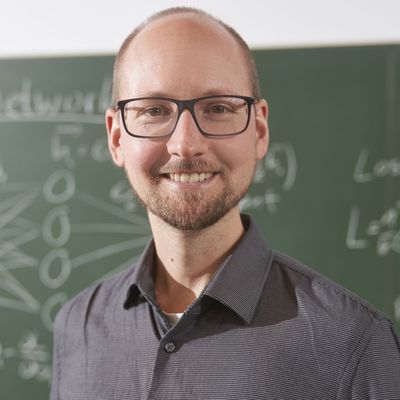
Pascal Friederich
KIT
Contact
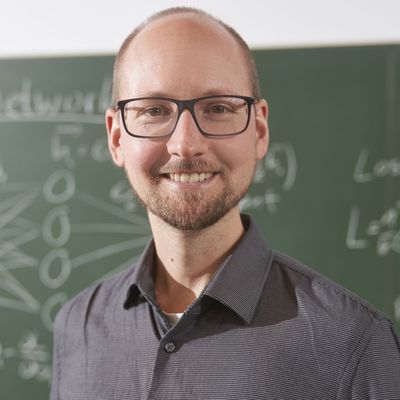
Our main research areas are
- Data-driven property prediction and design of materials and molecules
- Accelerated, machine learning based atomistic simulations of materials and molecular systems
- Machine learning methods for autonomous data analysis and decision-making in self-driving labs